Topology-Aware Network Optimization with Graph Neural Network
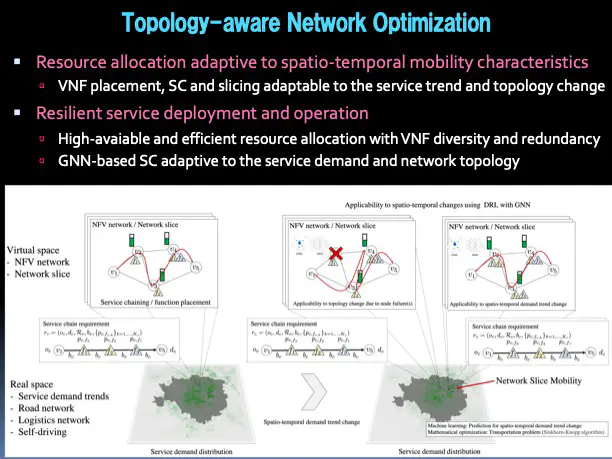
Network functions virtualization (NFV) enables a communication network to provide more flexible network services by replacing the traditional network appliances with generic hardware and executing the network functions virtually on them, which are called virtual network functions (VNFs). To realize a service chain from its requirements, the service chaining problem arises, which is a kind of combinational optimization problems. More specifically, the service chaining problem is finding a suitable service path from the origin to the destination such that the VNFs are executed at the intermediate node in the required order under the resource constraints. The service chaining problem is one of the resource allocation problems in the NFV network and belongs to the complexity class NP-hard. NFV network topologies may continuously change according to service chain demand trends. Since the changes in the virtual topology configuration affect the resource occupation on the physical network, we need to allocate network resources by considering the connectivity and characteristics of both the physical and virtual networks. In this research, considering the spatio-temporal mobility patterns, we aim to achieve both efficient network resource utilization and rapid service provisioning, and to realize service chaining that can adaptively follow changes in topologies by utilizing deep reinforcement learning and graph neural networks. In addition, we aim to achieve high-available and efficient VNF placement with VNF diversity and redundancy to ensure a resilient network service and realize the network slicing adaptive to the spatio-temporal mobility patterns.