Intelligent in-Network Training/Inference Mechanism
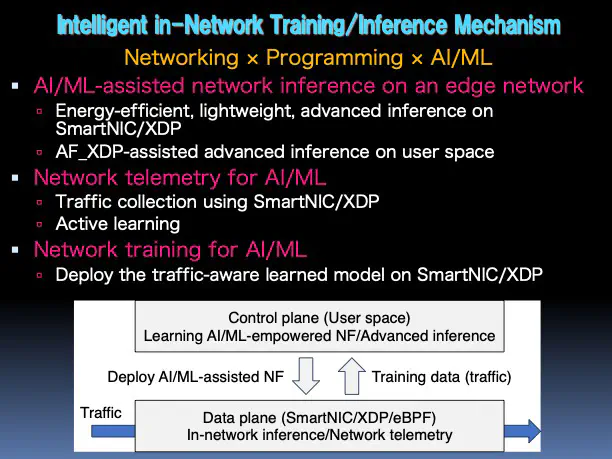
Recently, various things are connected via networks. In particular, traffic on networks must be handled appropriately to ensure the safe and continuous operation of generative artificial intelligence (AI), automated driving, and/or smart factories. Network softwarization and programmability, which have attracted much attention in recent years, will accelerate the integration of machine learning (ML) and AI and realize intelligent traffic processing. In particular, AI/ML has demonstrated capabilities that rival human intelligence in certain areas. The existing in-network inference allows the AI on dedicated devices to perform advanced traffic engineering on the core network designed to operate at high throughput. However, there are still concerns about the deployment of AI in the edge network from the viewpoint of capital and operating expenditure. In this research, we aim to establish an AI-empowered network inference mechanism using SmartNICs and XDPs, which can be deployed on general-purpose devices at low cost, in order to achieve energy-efficient, lightweight, and advanced traffic processing in edge environments. The proposed network inference mechanism requires a continuous cycle of efficient traffic collection, advanced learning that can follow traffic trends, and fast and advanced traffic classification. This research aims to establish the mechanism for smoothly executing these cycles through the integration of networking, programming, and AI, and to realize networking with power-saving, robustness, and intelligence capabilities.